Advancements in experimental protein structure determination have massively expanded the Protein Data Bank (PDB) filling in significant knowledge gaps regarding protein structure and function. However, experimental structures are often too low resolution for accurate proton placement. Protein acid-base chemistry is especially important for catalytic sites in protein-protein interactions and ligand binding sites. To accurately place protons in protein structures, we can use computational methods to consider the effects of pH on titratable residues (ASP, GLU, HIS, LYS) in protein structure. One such metric for determining pH effects is the pKa shifts of protein residues. Constant pH molecular dynamics-based methods for protein acid-base chemistry, while accurate, incur significant computational costs. On the other end, deep learning models offer pKa predictions at low computational cost but sacrifice conformational variance. Here, we introduce a novel method for fast pKa prediction for proteins using the polarizable AMOEBA force field. Leveraging statistical mechanics and side-chain optimization, we evaluate hundreds-of-thousands of protein conformations with varying titration states across a pH range to predict pKa. This method considers key effects of the protein’s environment (i.e. binding partners) that deep learning methods ignore. Here, residue pKa’s are predicted at a root-mean-squared-error (RMSE) of 0.78. This RMSE is on par with the available deep learning methods while also capturing the effects of binding partners on proton placement that are left out of the deep learning methods. This is highlighted in the example of the TBR1 transcription factor in complex with DNA. The deep learning models calculate the same pKa for TBR1 regardless of the presence of DNA. In direct contrast, this model correctly predicts the effect of DNA on the residues at the interaction interface. We can apply pH effects to protein structures and protein-protein complexes to better study their structure and function.
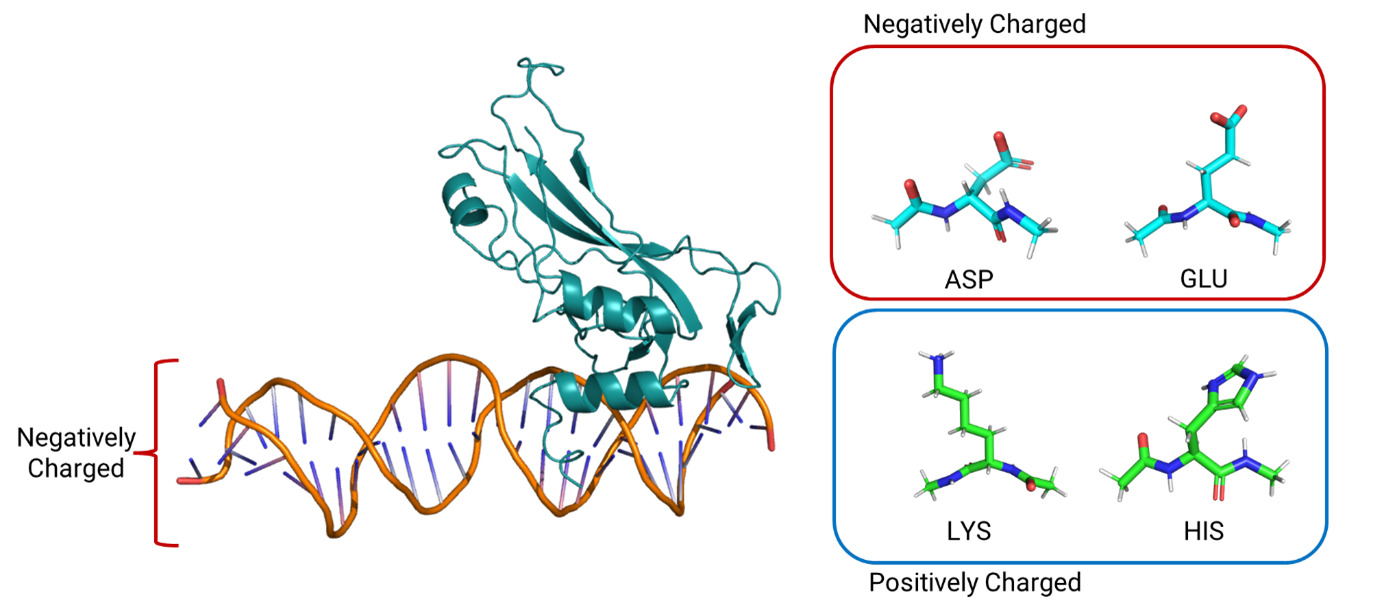