Despite advances in biomedical research, many diseases remain uncured. Surprisingly, about 90% of the human proteome is considered undruggable or lacks chemical probes. This gap presents both a challenge and an opportunity in drug discovery. In this talk, I will discuss our lab’s efforts to address this gap by developing databases and machine learning (ML) models aimed at comprehensive annotation of covalently druggable sites across the human proteome. Based on LigCys3D (a database of 1133 liganded cysteines in 778 proteins with X-ray crystal structure representations) and physics-based knowledge [1], we developed the tree and 3D-convolutional neural network (CNN) models to predict ligandable cysteine sites in monomer proteins as well as protein-protein interaction sites (PPIs), which achieved state-of-the-art performance (over 95% AUROC and 90% recall/precision) [2]. However, these first-generation models are limited by the protein bias of the Protein Data Bank (PDB) and the requirement of experimental structures as input. To address these limitations, we recently curated a new high-quality dataset comprised of cysteines liganded by drug-like molecules in chemoproteomic or co-crystallization experiments. The equivariant graph neural network models (GNNs) were then trained to predict cysteine-directed covalently druggable sites solely based on AlphaFold2 structure models. Finally, these models were subject to blinded tests using the unpublished chemoproteomic data. We will disseminate ABRIDGE (leverAge pdB, chemopRoteomics, and aI, to Discover druGgable sitEs), a new generation AI models to expand the druggable proteome, potentially unlocking new therapeutic targets for previously untreatable diseases.
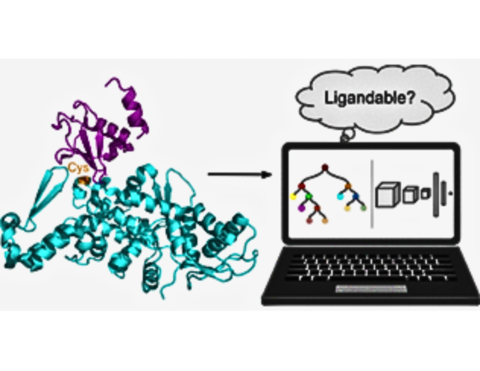
References:
Liu R, Yue Z, Tsai CC, and Shen J*. Assessing lysine and cysteine reactivities for designing targeted covalent kinase inhibitors. J Am Chem Soc 2019.
Ruibin Liu, Joseph Clayton, Mingzhe Shen, and Shen J*. Machine learning models for interrogating proteome-wide covalent ligandabilities directed at cysteines. JACS Au 2024.